How AI is improving credit scoring systems
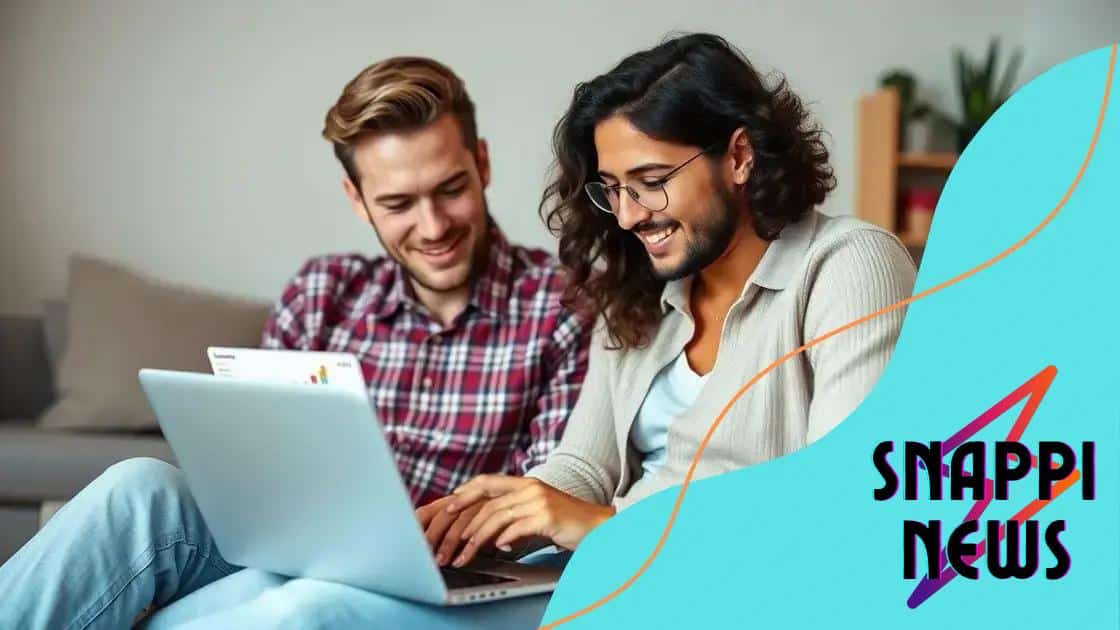
AI is improving credit scoring systems by using real-time data, machine learning, and alternative data sources to provide more accurate, fair assessments and reduce biases in lending.
How AI is improving credit scoring systems is a topic that many people are curious about. As technology advances, the way lenders assess creditworthiness is evolving, aiming for greater accuracy and fairness.
Understanding traditional credit scoring methods
Understanding traditional credit scoring methods is essential for grasping how AI is improving credit scoring systems. Credit scores have long been a primary tool for lenders to assess the risk involved in lending money. These scores are usually based on factors such as payment history, credit utilization, length of credit history, types of credit, and new credit inquiries.
To better understand this, we can break it down into several key components:
Key Factors Impacting Credit Scores
- Payment History: This accounts for a significant part of your credit score. Timely payments generally boost your score.
- Credit Utilization: Keeping credit card balances low compared to your limits can positively affect your score.
- Length of Credit History: A longer credit history may enhance your score, showing lenders you have experience managing credit.
- Types of Credit: A mix of revolving accounts (like credit cards) and installment loans (such as car loans) is often favorable.
These traditional scoring methods rely heavily on static data. For instance, individuals can struggle with low scores due to historical financial issues, even if their current situation is stable. This is where AI comes into play. By analyzing more extensive data sets, AI can provide a more nuanced picture of a borrower’s creditworthiness.
Furthermore, traditional models often fail to account for the rapid financial changes many experience today, such as job changes or economic fluctuations. With AI, lenders can adjust scoring mechanisms, ensuring they remain relevant and accurate.
The Limitations of Traditional Credit Scoring
While traditional models have served the industry for decades, they come with limitations:
- Inaccessibility: Many potential borrowers lack traditional credit histories, making it difficult to assess their risk.
- Inaccuracies: Errors in reporting can lead to unfairly low credit scores.
- Bias: Some traditional scoring methods may disadvantage minority groups or those with diverse financial backgrounds.
Thus, as we explore the role of AI, understanding these traditional scoring systems provides context for the evolution of credit assessment practices. Lenders are increasingly moving towards methods that incorporate machine learning and alternative data to enhance the accuracy and fairness of credit scoring.
The role of AI in data analysis
The role of AI in data analysis is pivotal in redefining how businesses assess and interpret information. With the vast amount of data generated daily, traditional methods can be slow and inefficient. Artificial intelligence aids in crunching numbers and providing insights rapidly and accurately.
One of the primary advantages of AI is its ability to analyze large datasets in real-time. This capability allows companies to make informed decisions based on current trends, rather than relying on outdated information. Machine learning algorithms can identify patterns and anomalies that would be challenging for humans to detect.
Improving Predictive Analytics
Predictive analytics is one area where AI shines brightly. By utilizing historical data, AI models can forecast future outcomes more accurately. For businesses, this means:
- Better risk assessment and management.
- Optimized marketing strategies tailored to customer needs.
- Enhanced inventory management to meet consumer demand.
Moreover, AI can automate the data preparation process, which often consumes much of analysts’ time. This automation allows teams to focus on interpretation and strategy, rather than data cleaning and formatting.
Natural Language Processing (NLP)
Another exciting aspect of AI in data analysis is its application in natural language processing. NLP enables machines to understand human language, allowing for deeper insights from textual data such as reviews and social media comments. By analyzing sentiments and topics within this data, companies can gain valuable feedback and adapt their strategies accordingly.
In summary, AI transforms data analysis from a time-consuming task to a swift, insightful process. The dynamic capabilities of AI empower businesses to leverage their data effectively, ensuring they remain competitive in an ever-evolving market.
Enhancing accuracy with machine learning
Enhancing accuracy with machine learning plays a crucial role in modern credit scoring systems. Traditional methods often rely on fixed algorithms that can overlook important factors or fail to adapt to changing financial landscapes. With machine learning, models evolve based on new data, leading to more precise assessments.
Machine learning algorithms can analyze a wider range of variables compared to traditional models. As they process vast amounts of data, these systems learn from patterns and correlations, which helps identify trends that humans might miss. For instance, they can evaluate not just credit history but also payment behavior and lifestyle indicators.
Benefits of Machine Learning in Credit Scoring
There are several key benefits to incorporating machine learning in credit scoring:
- Dynamic Learning: Systems improve over time as they gather more data, continuously refining their accuracy.
- Increased Data Utilization: Machine learning can analyze unstructured data, such as social media activities or payment behaviors.
- Personalized Scoring: Borrowers receive scores tailored to their unique financial profiles, making assessments fairer.
Through these advancements, machine learning helps lenders make well-informed decisions while reducing the risk of bias. This technology offers a significant opportunity to create a more equitable lending environment.
Moreover, machine learning models can quickly adapt to economic changes, such as recessions or booms. By recognizing shifts in data patterns, these systems maintain relevance, helping lenders respond efficiently to market dynamics.
Case Studies in Action
Several organizations have successfully implemented machine learning solutions in their credit scoring processes. These case studies demonstrate how accurately predictions can lead to better lending decisions:
- One bank used machine learning to reduce default rates by analyzing various transaction behaviors.
- Another organization improved its risk assessment model, allowing it to approve loans that traditional methods would have flagged as risky.
- A fintech company harnessed social data to enhance their scoring algorithms, resulting in broader access to credit.
These examples highlight the transformative power of machine learning in credit scoring. By enhancing accuracy, lenders can foster financial inclusion and drive fair lending practices across the industry.
Addressing biases in credit scoring
Addressing biases in credit scoring is vital for creating a fair financial system. Traditional credit scoring methods often rely on historical data that may reflect societal biases. This can lead to unfair treatment of certain groups, particularly minorities and low-income individuals.
Recognizing these biases is the first step toward improvement. For example, if a scoring system primarily uses data from well-off neighborhoods, it can disadvantage those from less affluent areas. This means that individuals with solid financial habits may receive low scores simply due to where they live.
Impact of Bias on Credit Decisions
To understand the impact of biases:
- Access to Credit: Many deserving borrowers might be denied loans because their scores are inaccurately low.
- Higher Interest Rates: Basing rates on flawed scores can lead to increased financial burdens for marginalized groups.
- Lack of Trust: If communities feel they are treated unfairly, they may become wary of financial institutions entirely.
By implementing AI and machine learning, we can analyze broader data sources that paint a more accurate picture of an individual’s creditworthiness. This approach helps include factors such as income stability and payment history, rather than just focusing on credit history.
Moreover, new technologies can help monitor and correct biases. For instance, organizations can regularly audit their scoring models to identify and mitigate any unfair practices. Active adjustments ensure that both lending practices and scoring reflect fair and equitable measures.
Strategies for Equitable Credit Scoring
To effectively address biases in credit scoring, organizations can adopt several strategies:
- Inclusive Data Collection: Collect diverse data to assess a wider range of credit behaviors.
- Model Testing: Regularly test algorithms for fairness and effectiveness by analyzing outcomes across various demographic groups.
- Community Outreach: Develop programs to educate underserved communities about credit and lending processes.
These strategies aim to ensure that credit scoring systems work for everyone, effectively leveling the playing field. By recognizing and addressing these biases, the financial industry can create a more inclusive future.
Future trends in credit scoring technology
Future trends in credit scoring technology are poised to reshape how lenders assess risk and make decisions. As advancements in technology continue to evolve, the way we understand and utilize credit scores will also change significantly.
One of the most exciting innovations is the growing integration of real-time data. Instead of relying solely on historical data, future systems may analyze ongoing financial behaviors, allowing for more accurate assessments. This approach will help lenders get a clearer picture of a borrower’s current financial status.
Adoption of Artificial Intelligence
Another trend is the increasing use of artificial intelligence (AI) and machine learning in credit scoring:
- Enhanced Accuracy: AI can analyze complex datasets to identify patterns that traditional models may miss, leading to better predictions.
- Personalized Assessments: Machine learning algorithms can tailor scoring models to individual borrowers, considering their unique circumstances.
- Continuous Improvement: As more data is inputted, AI systems can evolve and improve their predictive abilities over time.
In addition to AI, there is a push toward utilizing alternative data sources. These can include non-traditional information such as utility payments, rental histories, and social media activity. By broadening the scope of what is considered in a credit assessment, lenders can provide opportunities to those who may have been historically underserved.
Focus on Transparency and Fairness
The credit scoring industry is also expected to emphasize transparency and fairness:
- Clear Criteria: Future scoring models will aim to define clear criteria for how scores are calculated, allowing borrowers to understand and improve their scores.
- Bias Mitigation: As awareness of biases grows, scoring systems will work to minimize their impact, ensuring fair treatment across diverse populations.
- Consumer Control: Borrowers might gain more control over their data, allowing them to manage how their information is used in credit assessments.
As these trends emerge, the future of credit scoring technology looks promising. These advancements aim to create a more equitable credit landscape, ultimately allowing more individuals to access the credit they need to thrive.
FAQ – Frequently Asked Questions about AI in Credit Scoring
How does AI improve credit scoring accuracy?
AI analyzes large datasets quickly and identifies patterns that traditional models may miss, leading to more accurate assessments.
What is real-time data in credit scoring?
Real-time data allows lenders to assess a borrower’s current financial behavior, rather than just relying on historical data.
Why are alternative data sources important?
Alternative data sources, like rental payment histories and utility bills, help provide a more comprehensive view of a borrower’s creditworthiness.
How do biases affect credit scoring?
Biases can lead to unfair treatment of certain groups by relying on outdated data, which may not reflect a person’s true financial situation.