The role of machine learning in automating financial operations
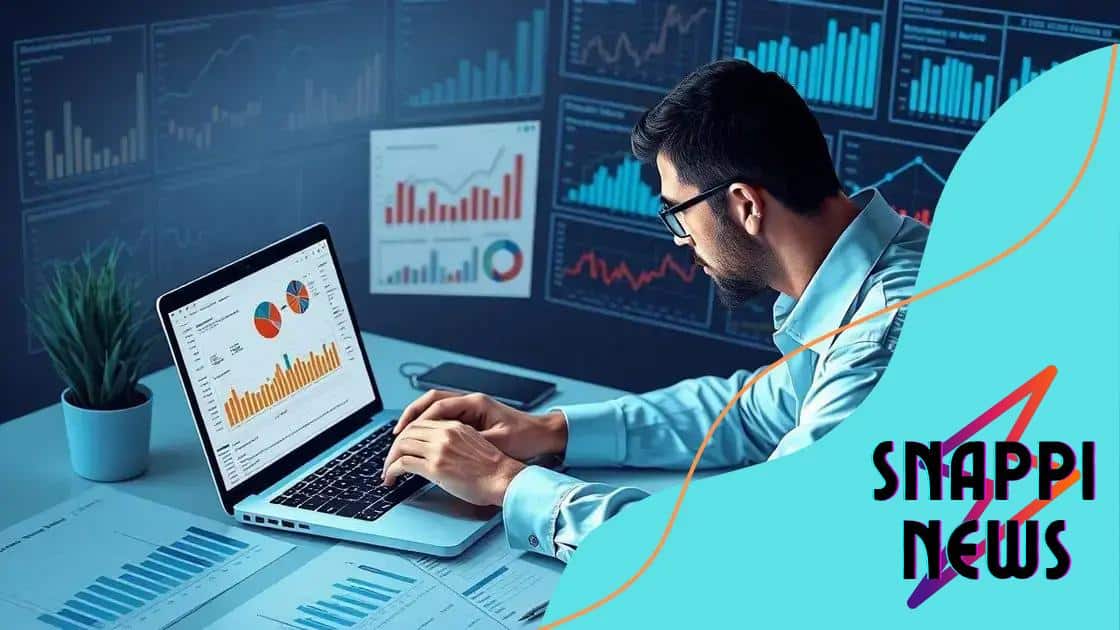
The role of machine learning in automating financial operations involves enhancing efficiency, reducing errors, and providing real-time insights through advanced data analysis, contributing significantly to the future of finance technology.
The role of machine learning in automating financial operations is gaining traction, and for good reason. Have you noticed how technology simplifies complex tasks? With the rise of machine learning, financial processes are not just faster; they’re smarter.
Understanding machine learning basics
Understanding machine learning basics is essential in today’s tech-driven world. Many people are curious about how this technology works and its impact on our lives. Machine learning is a fascinating field that allows computers to learn from data, improving their performance over time.
Machine learning is actually a branch of artificial intelligence. It focuses on developing algorithms that can analyze data, identify patterns, and make decisions with minimal human intervention. By using large volumes of data, machine learning models can identify trends and predict outcomes efficiently.
Key Concepts in Machine Learning
There are several important concepts to grasp in machine learning:
- Supervised Learning: This involves training a model on labeled data, where the input and output are known.
- Unsupervised Learning: In this method, the model learns from unlabeled data by finding patterns and structures.
- Reinforcement Learning: Here, an agent learns by interacting with an environment to maximize rewards.
Another crucial aspect is feature selection. It is the process of identifying the most important variables in your dataset to improve model accuracy. A well-chosen feature set can lead to better predictions and insights.
Furthermore, training and testing data are fundamental to creating a reliable model. The data is typically divided into two sets: one for training the model and another for testing its performance. This practice helps ensure that the model can generalize well to new data.
Applications in Real Life
The applications of machine learning are vast and varied. From predicting stock prices to analyzing customer behavior, its influence touches many aspects of modern life. Automated systems utilize machine learning to enhance user experiences, from personalized recommendations on streaming services to fraud detection in banking.
By understanding the basics of machine learning, one can appreciate its potential to innovate technology. Companies harness these models to optimize processes and gain valuable insights. This creates efficiency and leads to smarter decision-making.
Benefits of automation in finance
The benefits of automation in finance are extensive and transformative. Automation streamlines processes, reduces human error, and enhances efficiency in financial operations. As technology advances, organizations find innovative ways to integrate automation into their financial systems.
One major advantage is the ability to process data quickly. Automated systems can handle large volumes of transactions without getting tired or making mistakes. This leads to faster decision-making and ensures that important financial information is available in real-time.
Cost Savings
Another significant benefit is cost savings. By automating routine tasks, companies can allocate their resources more effectively. This means less money spent on manual work and more focus on strategic initiatives. Employees can shift their attention from mundane tasks to higher-value activities, driving productivity.
- Reduced labor costs: Fewer employees are needed for repetitive tasks.
- Minimized errors: Automation lowers the chances of mistakes that can be costly.
- Efficiency gains: Processes become quicker and more efficient.
Moreover, automation improves regulatory compliance. Financial institutions must adhere to strict regulations, and automated systems can help keep track of compliance requirements seamlessly. These systems can automatically generate reports and ensure timely submissions, reducing the risk of penalties or fines.
Enhanced customer experiences are another benefit. Automation allows for faster service delivery, such as quicker transaction processing and instant responses to customer queries. It facilitates personalized communication by analyzing customer data, leading to better engagement.
Data-Driven Insights
With automation, financial organizations gain access to valuable data-driven insights. Databases can analyze trends and identify patterns that humans might miss. This information enables businesses to make informed decisions, uncover new opportunities, and stay competitive in the market.
Overall, the benefits of automation in finance are compelling. As more companies adopt these technologies, they position themselves for growth and success in an increasingly competitive landscape. Embracing automation not only transforms operations but also enhances strategic focus, paving the way for innovation.
Real-world applications of machine learning
The real-world applications of machine learning are diverse and impactful. Businesses are increasingly adopting this technology to enhance their operations and achieve significant results. From healthcare to finance, machine learning is reshaping industries by providing innovative solutions.
In the healthcare sector, machine learning helps in diagnosing diseases and predicting patient outcomes. Algorithms analyze medical records and imaging data to assist doctors in making informed decisions. For example, machine learning models can identify tumors in radiology images faster than human specialists, improving accuracy and patient care.
Finance and Banking
In finance, the applications are equally transformative. Machine learning algorithms detect fraudulent transactions by monitoring patterns in users’ behavior. If an anomaly occurs, the system triggers alerts, minimizing losses for banks and customers.
- Risk assessment: Lenders use machine learning to evaluate the creditworthiness of individuals and businesses.
- Algorithmic trading: Firms leverage machine learning to execute trades based on market trends and data analysis.
- Customer support: Automated chatbots provide assistance to clients, streamlining service while enhancing user experience.
In the retail industry, machine learning enhances customer experiences through personalization. By analyzing shopping patterns, businesses can offer targeted promotions and recommendations. This leads to increased customer satisfaction and higher sales.
Transportation and Logistics
Transportation is also benefiting from machine learning. Ride-sharing apps utilize algorithms to optimize routes, ensuring shorter wait times for passengers. Logistics companies use machine learning to manage inventory and predict demand, resulting in more efficient supply chains.
Moreover, autonomous vehicles rely on machine learning to navigate their environments safely. Systems process information from sensors in real-time, making decisions that mimic human intuition. This application showcases the potential of machine learning to revolutionize transportation.
Overall, the real-world applications of machine learning demonstrate its versatility and power. As more industries adopt this technology, they unlock new possibilities for innovation and improvement. The impact of machine learning will continue to grow, making it an essential tool for the future.
Challenges of implementing machine learning
The challenges of implementing machine learning can be significant. While this technology offers many benefits, organizations often face obstacles when trying to integrate it into their operations. Understanding these challenges is essential for successful adoption.
One major issue is the quality of data. Machine learning models require large amounts of high-quality data to learn effectively. If the data is incomplete, outdated, or biased, it can lead to inaccurate predictions and outcomes. Companies must invest in proper data collection and management practices to address this challenge.
Skill Shortage
Another challenge is the skill shortage in the field. There are not enough qualified professionals who understand machine learning concepts and can develop effective models. Organizations often struggle to find talent with the right skills to implement and maintain machine learning systems.
- Training existing staff: Companies may need to provide training for their current employees to build the skills needed for machine learning projects.
- Hiring experts: Bringing in data scientists or machine learning engineers can be costly.
- Building a data team: Assembling a skilled team can be time-consuming and difficult.
Furthermore, integration with existing systems poses a challenge. Many organizations have legacy systems that are not designed to work with modern machine learning applications. This can lead to compatibility issues, delaying implementation and increasing costs.
Cost Considerations
The financial investment required for machine learning initiatives can also be daunting. Developing machine learning solutions often necessitates expensive software, hardware, and infrastructure upgrades. This may deter small and medium-sized businesses from pursuing machine learning projects despite the potential benefits.
Additionally, there are ethical considerations and regulatory concerns. Organizations must navigate data privacy laws and ensure that their machine learning applications do not inadvertently discriminate against individuals or groups. This adds another layer of complexity to the implementation process.
Despite these challenges, many organizations are finding ways to successfully implement machine learning. By understanding the common obstacles and planning accordingly, businesses can turn these challenges into opportunities for growth and innovation.
Future trends in financial technology
The future trends in financial technology are exciting and rapidly evolving. As technology continues to advance, finance is becoming more digital and efficient. One major trend is the rise of blockchain technology. This decentralized system offers enhanced security for transactions and greater transparency for users. Financial institutions are exploring how to implement blockchain in various applications, such as cross-border payments and smart contracts.
Another trend is the growing use of artificial intelligence (AI) and machine learning in finance. These technologies help companies analyze vast amounts of data to make better decisions. For instance, AI can enhance customer service through chatbots that provide instant support or even develop more personalized financial products.
Digital Banking
Digital banking is also transforming the finance industry. Traditional banks are adopting digital-first strategies to meet customer expectations for convenience and efficiency. Many people now prefer banking through mobile apps instead of visiting branches. This shift encourages banks to enhance their digital offerings and streamline operations.
- Mobile payment solutions: Services like Apple Pay and Google Pay continue to gain popularity, making transactions easier for consumers.
- Peer-to-peer lending: Platforms that connect borrowers directly with investors are changing how people access loans.
- Automated wealth management: Robo-advisors are rising, providing investment services with minimal human interaction.
Regulatory technology, or RegTech, is another key aspect of future trends. As regulations become more complex, technology will play a vital role in helping financial institutions comply with legal requirements efficiently. By automating compliance processes, organizations can reduce risk and lower costs.
Cybersecurity Innovations
As the digital landscape grows, cybersecurity becomes increasingly important. Financial companies are investing in advanced security measures to protect sensitive data. Innovations in security protocols are essential to avoid breaches and maintain customer trust.
In summary, the future trends in financial technology point toward a more interconnected and efficient financial system. By embracing these innovations, financial institutions can create better experiences for their customers, improve security, and drive overall growth in the industry.
FAQ – Frequently Asked Questions about Future Trends in Financial Technology
What is blockchain technology and how does it benefit finance?
Blockchain technology enhances transaction security and transparency, making it easier to track and verify transactions.
How can artificial intelligence (AI) improve customer service in finance?
AI can streamline responses through chatbots and analyze customer data to provide personalized services, improving overall customer satisfaction.
What role does digital banking play in modern finance?
Digital banking provides convenience for users by enabling online services, mobile access, and faster transactions, aligning with customer preferences.
What is RegTech and why is it important?
RegTech, or regulatory technology, automates compliance processes to help financial institutions adhere to rules efficiently, minimizing regulatory risks.